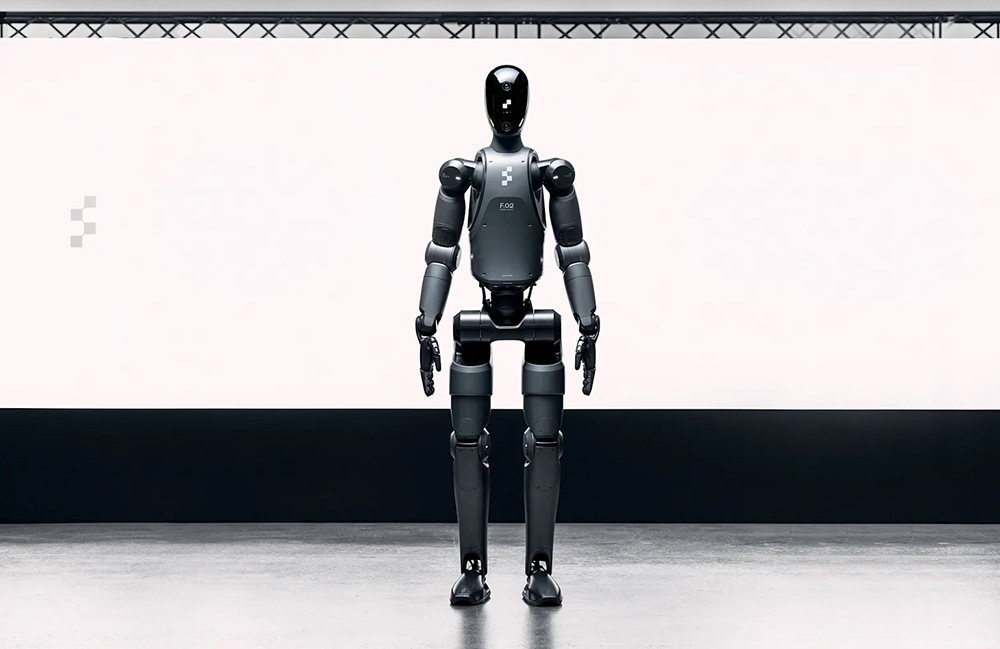
Determine is constructing the Helix mannequin and a knowledge engine to allow a fleet of humanoid robots to study logistics duties. Supply: Determine AI
Determine AI Inc. this week launched a real-world software for its humanoid robots and Helix mannequin: package deal manipulation for triage in logistics.
“This activity calls for human-level velocity, precision, and flexibility, pushing the boundaries of pixels-to-actions discovered manipulation,” stated the Sunnyvale, Calif.-based firm.
On the core of this software is Helix, Determine’s internally designed vision-language-action (VLA) mannequin. Introduced simply final week, this mannequin unifies notion, language understanding, and discovered management.
Humanoid addresses logistics use case
This activity presents a number of key challenges, famous Determine AI. Packages are available in all kinds of sizes, shapes, weights, and rigidity – from inflexible bins to deformable luggage, making them troublesome to copy in simulation.
The Determine 02 humanoid robotic should decide the optimum second and methodology for greedy the transferring object and reorienting every package deal to reveal the label. Moreover, it wants to trace the dynamic move of quite a few packages on a constantly transferring conveyor and preserve a excessive throughput.
Because the setting can by no means be absolutely predictable, the system should have the ability to self-correct. Addressing these challenges isn’t solely a key software of Determine’s enterprise; it additionally yielded generic new enhancements to Helix System 1 that each one different use instances now profit from, the corporate stated.
Helix visible illustration improves
Determine AI claimed that its system now has a wealthy 3D understanding of its setting, enabling extra exact depth-aware movement. Whereas its earlier System 1 relied on monocular visible enter, the brand new System 1 makes use of a stereo imaginative and prescient spine coupled with a multiscale characteristic extraction community to seize wealthy spatial hierarchies.
Slightly than feeding picture characteristic tokens from every digicam independently, options from each cameras are merged in a multiscale stereo community earlier than being tokenized, defined the corporate. This retains the general variety of visible tokens fed to Determine’s cross-attention transformer fixed and avoids computational overhead.
The multiscale options enable the system to interpret high quality particulars in addition to broader contextual cues, which collectively contributing to extra dependable management from imaginative and prescient, Determine stated.
Register immediately to save lots of 40% on convention passes!
Getting ready for deployments at scale
Deploying a single coverage on many robots requires addressing distribution shifts within the remark and motion areas on account of small particular person robotic {hardware} variations. These embrace sensor-calibration variations (affecting enter observations) and joint response traits (affecting motion execution), which might influence coverage efficiency if not correctly compensated for, stated Determine AI.
Particularly with a high-dimensional, whole-upper-body motion area, conventional handbook robotic calibration doesn’t scale over a fleet of robots. As an alternative, Determine trains a visible proprioception mannequin to estimate the 6D poses of finish effectors solely from every robotic’s onboard visible enter.
This on-line “self-calibration” permits robust cross-robot coverage switch with minimal downtime, the corporate stated.
Through the use of the discovered calibration and visible proprioception module, Determine was in a position to apply the identical coverage, initially educated on a single robotic’s information, to a number of extra robots. Regardless of variations in sensor calibration and small {hardware} variations, the system maintained a comparable degree of manipulation efficiency throughout all platforms, it asserted.
Determine stated this consistency demonstrated the effectiveness of discovered calibration in mitigating covariate shifts, successfully lowering the necessity for tedious per-robot recalibration and making large-scale deployment extra sensible.
Information curation and dashing up manipulation
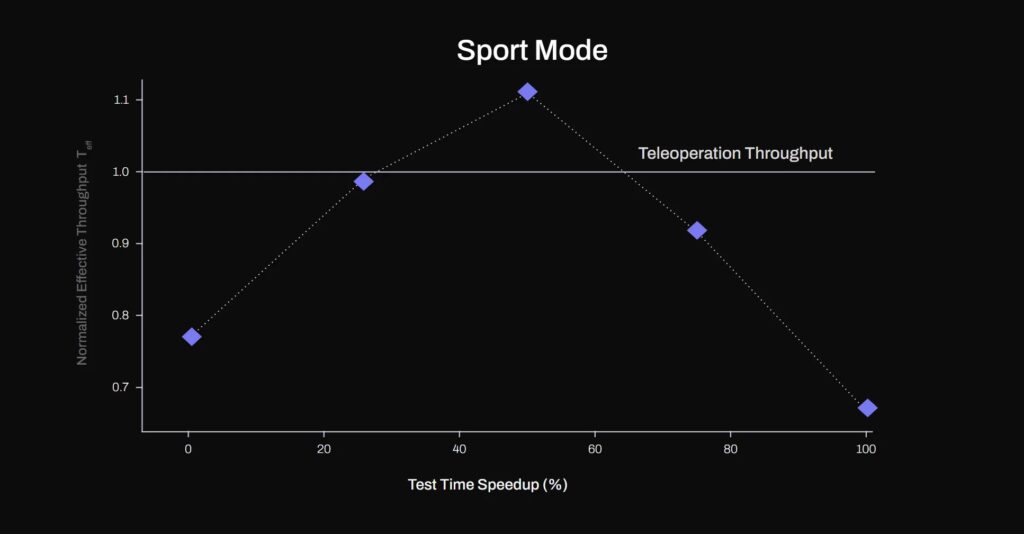
On the info aspect, Determine stated it took specific care in filtering human demonstrations, excluding the slower, missed, or failed ones. Nevertheless, it intentionally stored demonstrations that naturally included corrective conduct when the failure that prompted the correction was deemed on account of environmental stochasticity somewhat than operator error.
Working intently with teleoperators to refine and uniformize manipulation methods additionally resulted in vital enhancements, the corporate stated.
Along with uniformizing manipulation methods, Determine has additionally labored to finally transcend human manipulation velocity.
It utilized a easy test-time approach that yielded faster-than-demonstrator discovered conduct. Determine interpolated the coverage motion chunk output, which referred to as “Sport Mode.” Its System 1 insurance policies output motion “chunks,” representing a collection of robotic actions at 200hz.
As an example, the corporate stated it may well obtain a 20% test-time speedup, with none modifications to the coaching process, by linearly re-sampling an motion chunk of [T x action_dim]—representing an T-millisecond trajectory—to a shorter [0.8 * T x action_dim] trajectory, then executing the shorter chunk on the unique 200 Hz management fee.
Dashing up the coverage execution through linear re-sampling or “sport mode” was efficient as much as a 50% velocity up, the corporate stated. That is possible rendered doable by the excessive temporal decision (200Hz) of the motion outputs chunks.
Nevertheless, when going past 50% velocity up, the efficient throughput began to drop considerably as motions change into too imprecise, and the system wanted to be reset ceaselessly, reported Determine. The corporate discovered that with a 50% velocity enhance, the coverage achieved quicker object dealing with in contrast with the skilled trajectories it’s educated on ( T_eff>1).
Determine AI shares Helix outcomes up to now
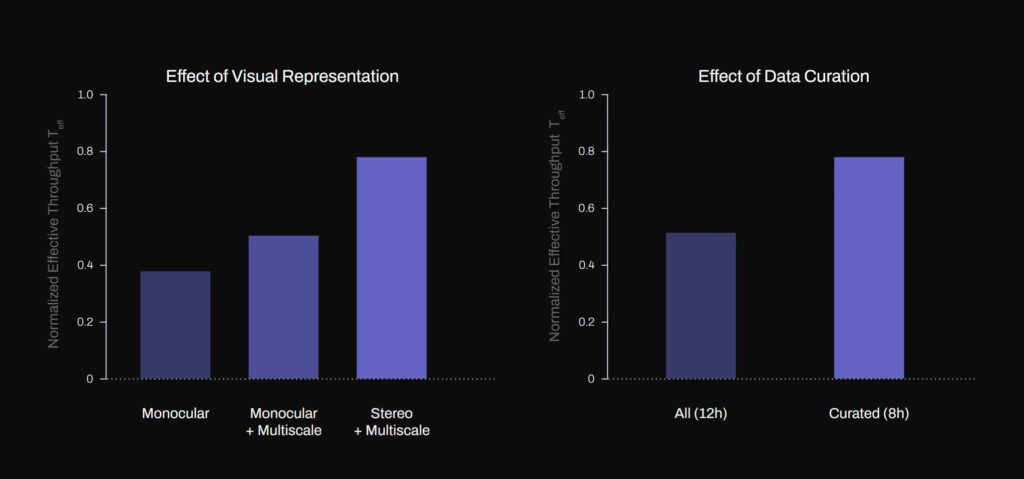
Determine measured the system’s efficiency utilizing the normalized efficient throughput T_eff, which represents how briskly packages are dealt with in contrast with the demonstration information it’s educated on. This takes under consideration any time spent resetting the system if vital.
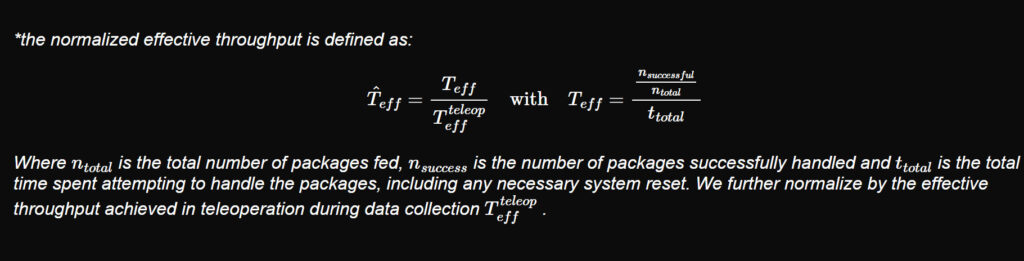
For instance, T_eff > 1.1 represents a manipulation velocity 10% quicker than the skilled trajectory collected for coaching. Determine AI stated it discovered that each the multiscale characteristic extraction and implicit stereo enter might considerably enhance system efficiency.
The corporate additionally famous the improved robustness to varied package deal sizes when including stereo. The stereo mannequin achieves a 60% enhance in throughput over non-stereo baselines.
As well as, Determine discovered that the stereo-equipped S1 can generalize to flat envelopes that the system was by no means educated on.
The corporate additionally discovered that for a single use case, information high quality and consistency mattered way more than information amount. Its outcomes confirmed {that a} mannequin educated with curated, high-quality demonstrations achieved 40% higher throughput regardless of being educated with one-third much less information.
Determine AI concluded that it has discovered how a high-quality dataset, mixed with architectural refinements akin to stereo multiscale imaginative and prescient, on-line calibration, and a test-time velocity up, can obtain faster-than-demonstrator dexterous robotic manipulation in a real-world logistics triaging state of affairs.
Its system did this all whereas utilizing comparatively modest quantities of demonstration information, stated the corporate. Determine stated Helix exhibits the potential for scaling end-to-end visuo-motor insurance policies to advanced industrial functions the place velocity and precision are essential.