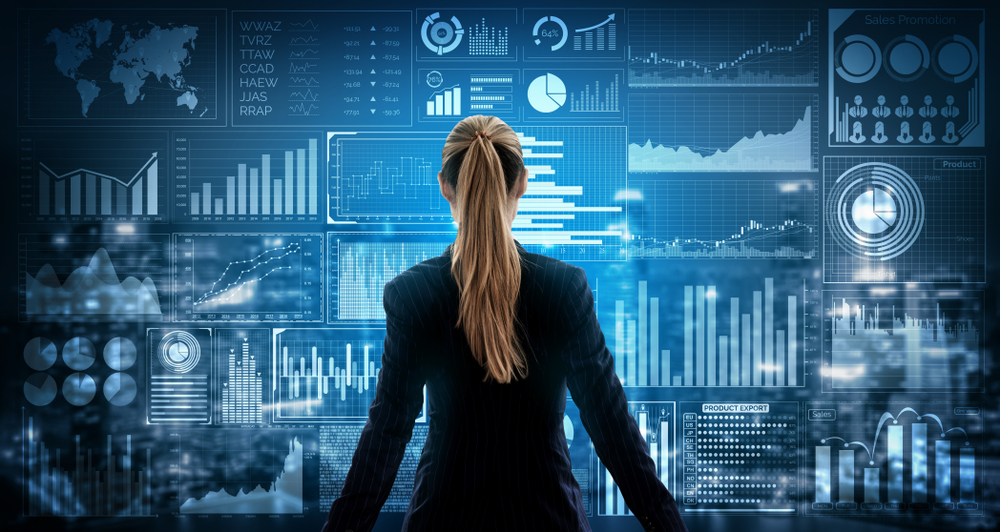
(Summit Artwork Creations/Shutterstock)
Development in enterprise AI’s early years has largely been outlined by experimentation, with companies testing varied fashions and seeing speedy enhancements. Nonetheless, as the highest LLMs’ capabilities converge, AI brokers change into extra prevalent and domain-specific small language fashions acquire momentum, knowledge methods are more and more the deciding issue driving AI success.
Sadly, most companies’ knowledge architectures at the moment have clear shortcomings. Seventy-two % of organizations cite knowledge administration as one of many prime challenges stopping them from scaling AI use circumstances. Particularly, three particular knowledge administration challenges persistently rise to the floor for knowledge leaders as they work to deploy AI.
Managing Skyrocketing Knowledge Volumes
Enterprise knowledge’s progress and rising complexity has overwhelmed conventional infrastructure and created bottlenecks that restrict AI initiatives. Organizations not solely have to retailer large quantities of structured, semi-structured and unstructured knowledge, however this knowledge additionally must be processed to be helpful to AI purposes and RAG workloads.
Superior {hardware} like GPUs course of knowledge a lot sooner and extra cost-effectively than beforehand potential, and these advances have fueled AI’s breakthroughs. But, the CPU-based knowledge processing software program most companies have in place can’t reap the benefits of these {hardware} advances. Whereas these programs served their objective for extra conventional BI utilizing structured knowledge, they will’t sustain with right now’s mountains of unstructured and semi-structured knowledge making it very gradual and costly for enterprises to leverage the vast majority of their knowledge for AI.
As AI’s knowledge wants have change into clearer, knowledge processing developments have begun to account for the dimensions and complexity of recent workloads. Profitable organizations are reevaluating the programs they’ve in place and implementing options that enable them to reap the benefits of optimized {hardware} like GPUs.
Overcoming Knowledge Silos
Structured, semi-structured, and unstructured knowledge have traditionally been processed on separate pipelines that silo knowledge, leading to over half of enterprise knowledge being siloed. Combining knowledge from completely different pipelines and codecs is advanced and time-consuming, slowing real-time use circumstances like RAG and hindering AI purposes that require a holistic view of information.
For instance, a retail buyer assist chatbot must entry, course of and be a part of collectively knowledge from varied sources to efficiently reply to buyer queries. These sources embody structured buyer buy info that’s typically saved in a knowledge warehouse and optimized for SQL queries, and on-line product suggestions that’s saved in unstructured codecs. With conventional knowledge architectures, becoming a member of this knowledge collectively is advanced and costly, requiring separate processing pipelines and specialised instruments for every knowledge sort.
Thankfully, it’s changing into simpler to remove knowledge silos. Knowledge lakehouses have change into more and more widespread, permitting companies to retailer structured, semi-structured, and unstructured knowledge of their authentic codecs in a unified setting. This eliminates the necessity for separate pipelines and will help AI purposes acquire a extra holistic view of information.
Nonetheless, most incumbent knowledge processing programs have been designed for structured knowledge, making it gradual and costly to course of the various knowledge lakehouses retailer. Organizations are discovering that with a view to lower the fee and latency of AI purposes and allow real-time use circumstances, they should transfer past lakehouses and unify their whole knowledge platform to deal with all varieties of knowledge.
Guaranteeing Knowledge High quality
The early thesis with LLM improvement was that extra knowledge equals larger and higher fashions, however this scaling regulation is more and more being questioned. As LLM development plateaus, a larger onus falls on the contextual knowledge AI prospects have at their very own disposal.
Nonetheless, guaranteeing this knowledge is high-quality is a problem. Widespread knowledge high quality points embody knowledge saved in conflicting codecs that confuse AI fashions, stale information that result in outdated choices, and errors in knowledge entry that trigger inaccurate outputs.
Gartner estimates poor knowledge high quality is a key purpose 30% of inside AI initiatives are deserted. Present strategies for guaranteeing knowledge high quality are additionally inefficient, as 80% of information scientists’ time is spent accessing and getting ready knowledge. On prime of that, a big share of this time is spent cleansing uncooked knowledge.
To make sure knowledge high quality for AI purposes, companies ought to outline clear knowledge high quality metrics and requirements throughout the group to make sure consistency, undertake knowledge high quality dashboards and profiling instruments that flag anomalies, and implement libraries that assist standardize knowledge codecs and implement consistency.
Whereas AI presents companies unbelievable alternatives to innovate, automate, and acquire a aggressive edge, success hinges on having a sturdy knowledge technique and rethinking present knowledge architectures. By addressing the challenges of managing skyrocketing knowledge volumes, unifying knowledge pipelines, and guaranteeing knowledge high quality, organizations can lay a stable basis for AI success.
In regards to the writer: Rajan Goyal is co-founder and CEO of DataPelago, which is creating a common knowledge processing engine to unite large knowledge, superior analytics, and AI. Goyal has a confirmed observe report of main merchandise from inception to multi-billion greenback income. With 50+ patents and experience in pioneering DPU structure, Rajan has held key roles at Cisco, Oracle, Cavium, and Fungible, the place he served as CTO. He holds levels from the Thapar Institute of Engineering and Know-how and Stanford College.
Associated Gadgets:
Knowledge High quality Bought You Down? Thank GenAI
Knowledge High quality Getting Worse, Report Says
DataPelago Unveils Common Engine to Unite Large Knowledge, Superior Analytics, and AI Workloads